This is about the matrix balancing problem.
We have three sets of data:
- A matrix with with entries \(\color{darkblue}A^0_{i,j}\ge 0\).
- Row- and column-totals \(\color{darkblue}r_i\) and \(\color{darkblue}c_j\).
The \(\color{darkblue}A^0\) matrix is collected from different sources than the row- and column-totals. So the matrix elements don't sum up to our totals. The problem is finding a nearby matrix \(\color{darkred}A\), so the row and column totals are obeyed. In addition, we want to preserve the sparsity pattern of \(\color{darkblue}A^0\): zeros should stay zero. And also: we don't want to introduce negative numbers (preserve the signs). More formally:
Matrix Balancing Problem |
\[\begin{align}\min\>&{\bf{dist}}(\color{darkred}A,\color{darkblue}A^0)\\ & \sum_i \color{darkred}A_{i,j} = \color{darkblue}c_j && \forall j\\ & \sum_j \color{darkred}A_{i,j} = \color{darkblue}r_i && \forall i \\&\color{darkred}A_{i,j}=0 &&\forall i,j|\color{darkblue}A^0_{i,j}=0\\ &\color{darkred}A_{i,j}\ge 0 \end{align} \] |
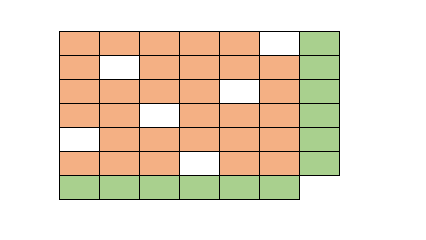 |
Approximate the matrix subject to row- and column-sum constraints |
Data
I invented some (random) data so we can scale the problem up to any size. The data generation algorithm is:
- Generate the sparsity pattern of the matrix
- Populate the non-zero elements of the matrix.
- Calculate the row and column sums.
- Perturb the row and column sums. I used a multiplication with a random number. This makes sure things stay positive.
Maintaining feasibility can be a bit finicky, especially if the matrix is sparse. Somehow it helps to perturb the totals instead of the matrix itself.
I used a \(1000 \times 1000\) matrix in the experiments below.
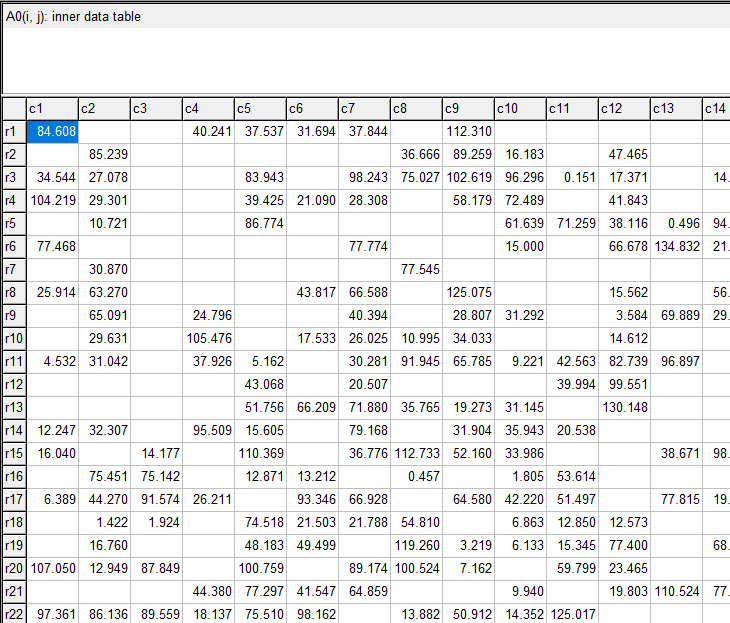 |
Partial view of the generated matrix \(\color{darkblue}A^0\). |
This is not a small problem, with about half a million (non-linear) variables. But this is still way smaller than the problems we may see in practice, especially when we regionalize.
The RAS algorithm
The RAS algorithm for bi-proportional fitting [1] is extremely popular. It is very simple, fast, and converges often in less than 10 iterations. The structure is as follows:
RAS Algorithm |
\(\color{darkred}A_{i,j} := \color{darkblue}A^0_{i,j}\)
loop
# row scaling
\(\color{darkred}\rho_i := \displaystyle\frac{\color{darkblue}r_i}{\sum_j \color{darkred}A_{i,j}}\)
\(\color{darkred}A_{i,j} := \color{darkred}\rho_i\cdot \color{darkred}A_{i,j}\)
# column scaling
\(\color{darkred}\sigma_j := \displaystyle\frac{\color{darkblue}c_j}{\sum_i \color{darkred}A_{i,j}}\)
\(\color{darkred}A_{i,j} := \color{darkred}\sigma_j\cdot \color{darkred}A_{i,j}\)
until stopping criterion met
|
We can stop when an iteration limit is reached or when the quantities \(\rho_i\) and \(\sigma_j\) are sufficiently close to 1.0. In the GAMS code below, we stop either on iterations or on a converge measure.
Below I show the convergence speed by printing the maximum deviation from 1.0 for \(\rho_i\) and \(\sigma_j\). Or in different words: \[\left\|{{\rho-1}\atop{\sigma-1}}\right\|_{\infty}\]
---- 94 PARAMETER trace RAS convergence
||rho-1|| ||sigm-1|| ||both||
iter1 0.04499855 0.05559031 0.05559031
iter2 0.00233051 0.00012370 0.00233051
iter3 0.00000958 0.00000059 0.00000958
iter4 0.00000004 3.587900E-9 0.00000004
---- 95 RAS converged.
PARAMETER niter = 4.000 iteration number
This demonstrates excellent performance. Actually, it is quite phenomenal, when considering we are dealing with half a million non-linear variables \(\color{darkred}A_{i,j}\).
Entropy Maximization
The following Entropy based optimization model will give the same solutions as the RAS algorithm above.
The original entropy maximization problem has an objective of the form: \[\max\>H=-\sum_{i,j} b_{i,j} \ln b_{i,j}\] For our RAS emulation, we use a so-called cross entropy form: \[\min\>\sum_{i,j} b_{i,j} \ln \frac{b_{i,j}}{a_{i,j}}\] Our model becomes:
Cross-Entropy Optimization Problem |
\[\begin{align}\min&\sum_{i,j|A^0_{i,j}\gt 0}\color{darkred}A_{i,j} \cdot \ln \frac{\color{darkred}A_{i,j}}{\color{darkblue}A^0_{i,j}}\\ & \sum_i \color{darkred}A_{i,j} = \color{darkblue}c_j && \forall j\\ & \sum_j \color{darkred}A_{i,j} = \color{darkblue}r_i && \forall i \\&\color{darkred}A_{i,j}=0 &&\forall i,j|\color{darkblue}A^0_{i,j}=0\\ &\color{darkred}A_{i,j}\ge 0 \end{align} \] |
To protect the logarithm, I added a small lower bound on \(\color{darkred}A\): \(\color{darkred}A_{i,j} \ge \text{1e-5}\).
Here are some results:
---- 222 PARAMETER report timings of different solvers
obj iter time
RAS alg -7720.083 4.000 0.909
CONOPT -7720.083 124.000 618.656
IPOPT -7720.083 15.000 171.602
IPOPTH -7720.083 15.000 25.644
KNITRO -7720.083 10.000 65.062
MOSEK -7720.051 13.000 11.125
Notes:
- The objective in the RAS algorithm was just an evaluation of the objective function after RAS was finished.
- In the GAMS model, we skip all zero entries. We exploit sparsity.
- CONOPT is an active set algorithm and is not really very well suited for this kind of model. The model has a large number of super-basic variables. (Super-basic variables are non-linear variables between their bounds). CONOPT does better when a significant fraction of the non-linear variables are at their bound. In this model, all variables are non-linear and are between their bounds.
- IPOPTH is the same as IPOPT but with a different linear algebra library (Harwell's MA27 instead of MUMPS). That has quite some impact on solution times.
- MOSEK is the fastest solver, but I needed to add an option: MSK_DPAR_INTPNT_CO_TOL_REL_GAP = 1.0e-5. This may need a bit more fine-tuning. Without this option, MOSEK would terminate with a message about a lack of progress. I assume it gets a little bit in numerical problems.
The precise messages (without using this option) are:
Interior-point solution summary
Problem status : UNKNOWN
Solution status : UNKNOWN
Primal. obj: -7.7200665399e+03 nrm: 3e+04 Viol. con: 3e-04 var: 0e+00 cones: 5e-05
Dual. obj: -7.7200934976e+03 nrm: 1e+00 Viol. con: 0e+00 var: 6e-10 cones: 0e+00
Return code - 10006 [MSK_RES_TRM_STALL]: The optimizer is terminated due to slow progress.
- MOSEK is not a general-purpose NLP solver. Older versions of MOSEK contained a general nonlinear convex solver, but this has been dropped. We have to use an exponential cone equation [4] for this entropy problem. This equation looks like: \[x_0 \ge x_1 \exp(x_2/x_1)\>\>\>\>\>\>\>\>x_0,x_1 \ge 0\] To use this equation we need to reformulate things a bit. This is a little bit more work, requires a bit more thought, and the resulting model is not as natural or obvious as the other formulations.
- The difference between the CONOPT solution and RAS solution for \(\color{darkred}A\) is small:
---- 148 PARAMETER adiff = 1.253979E-7 max difference between solutions
This confirms we have arrived at the same solution. For the other solvers, I only compared the optimal objective function value.
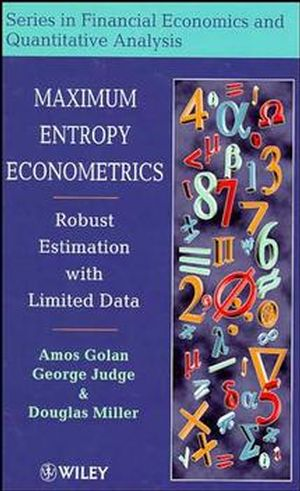 |
Entropy Models [2] |
References
- Iterative Proportional Fitting, https://en.wikipedia.org/wiki/Iterative_proportional_fitting
- Amos Golan. George Judge and Douglas Miller, Maximum Entropy Econometrics, Robust Estimation with Limited Data, Wiley, 1996
- McDougall, Robert A., "Entropy Theory and RAS are Friends" (1999). GTAP Working Papers. Paper 6.
- Dahl, J., Andersen, E.D., A primal-dual interior-point algorithm for nonsymmetric exponential-cone optimization. Math. Program. 194, 341–370 (2022).
Appendix: GAMS Model
$ontext
Matrix
Balancing:
RAS vs
Entropy Optimization
$offtext
*---------------------------------------------------------------
* size of the matrix
*---------------------------------------------------------------
set
i 'rows' /r1*r1000/
j 'columns'
/c1*c1000/
;
*---------------------------------------------------------------
* random sparse data
*---------------------------------------------------------------
Set P(i,j) 'sparsity pattern';
P(i,j) = uniform(0,1)<0.5;
Parameter A0(i,j) 'inner data table';
A0(p) = uniform(0,100);
Parameter
r(i) 'known row sums'
c(j) 'known column sums'
;
r(i) = sum(p(i,j), A0(p));
c(j) = sum(p(i,j), A0(p));
*
* perturb A0
*
A0(p) = uniform(0.5,1.5)*A0(p);
*---------------------------------------------------------------
* RAS
*---------------------------------------------------------------
set iter 'max RAS iterations' /iter1*iter50/;
Scalars
tol 'tolerance' /1e-6/
niter 'iteration
number'
diff1 'max(|rho-1|)'
diff2 'max(|sigma-1|)'
diff 'max(diff1,diff2)'
t 'time'
;
parameters
A(i,j) 'updated matrix using RAS method'
rho(i) 'row scaling'
sigma(j) 'column scaling'
trace(iter,*) 'RAS convergence'
;
t = timeElapsed;
A(i,j) = A0(i,j);
loop(iter,
niter = ord(iter);
*
* step 1 row scaling
*
rho(i) = r(i)/sum(j,A(i,j));
A(i,j) = rho(i)*A(i,j);
*
* step 2 column scaling
*
sigma(j) = c(j)/sum(i,A(i,j));
A(i,j) = A(i,j)*sigma(j);
*
* converged?
*
diff1 = smax(i,abs(rho(i)-1));
diff2 = smax(j,abs(sigma(j)-1));
diff = max(diff1,diff2);
trace(iter,'||rho-1||') = diff1;
trace(iter,'||sigm-1||') = diff2;
trace(iter,'||both||') = diff;
break$(diff < tol);
);
option trace:8; display trace;
if(diff < tol,
display "RAS converged.",niter;
else
abort "RAS did not converge";
);
t = timeElapsed-t;
parameter report 'timings of different solvers';
report('RAS alg','obj') = sum(p,A(p)*log(A(p)/A0(p)));
report('RAS alg','iter') = niter;
report('RAS alg','time') = t;
display report;
*---------------------------------------------------------------
* Entropy Model
*---------------------------------------------------------------
variable z 'objective';
positive variable A2(i,j) 'updated table';
* initial point
A2.l(p) = A0(p);
* protect log
A2.lo(p) = 1e-5;
equations
objective 'cross-entropy
objective'
rowsum(i) 'row totals'
colsum(j) 'column
totals'
;
objective.. z =e= sum(p,A2(p)*log(A2(p)/A0(p)));
rowsum(i).. sum(P(i,j), A2(p)) =e= r(i);
colsum(j).. sum(P(i,j), A2(p)) =e= c(j);
model m1 /all/;
option threads=0,
nlp=conopt;
solve m1
minimizing z using nlp;
*---------------------------------------------------------------
* Compare results
* ||A2-A||
*---------------------------------------------------------------
scalar adiff 'max difference between solutions';
adiff = smax((i,j),abs(A2.l(i,j)-A(i,j)));
display adiff;
*---------------------------------------------------------------
* Try different solvers
*---------------------------------------------------------------
report('CONOPT','obj') = z.l;
report('CONOPT','iter') = m1.iterusd;
report('CONOPT','time') = m1.resusd;
A2.l(p) = 0;
option nlp=ipopt;
solve m1
minimizing z using nlp;
report('IPOPT','obj') = z.l;
report('IPOPT','iter') = m1.iterusd;
report('IPOPT','time') = m1.resusd;
A2.l(p) = 0;
option nlp=ipopth;
solve m1
minimizing z using nlp;
report('IPOPTH','obj') = z.l;
report('IPOPTH','iter') = m1.iterusd;
report('IPOPTH','time') = m1.resusd;
A2.l(p) = 0;
option nlp=knitro;
solve m1
minimizing z using nlp;
report('KNITRO','obj') = z.l;
report('KNITRO','iter') = m1.iterusd;
report('KNITRO','time') = m1.resusd;
display report;
*---------------------------------------------------------------
* for mosek we use the exponential cone
*---------------------------------------------------------------
positive variable x1(i,j);
variable x3(i,j);
a2.l(p) = 1;
equations
expcone(i,j) 'exponential cone'
obj2 'updated
objective'
defx1 'x1 =
a0'
;
obj2.. z =e= -sum(p,x3(p));
expcone(p).. x1(p) =g= a2(p)*exp(x3(p)/a2(p));
defx1(p).. x1(p) =e= a0(p);
$onecho > mosek.opt
MSK_DPAR_INTPNT_CO_TOL_REL_GAP = 1.0e-5
$offecho
model m2 /obj2,expcone,rowsum,colsum,defx1/;
option nlp=mosek;
m2.optfile=1;
solve m2
minimizing z using nlp;
report('MOSEK','obj') = z.l;
report('MOSEK','iter') = m2.iterusd;
report('MOSEK','time') = m2.resusd;
display report;
|
No comments:
Post a Comment